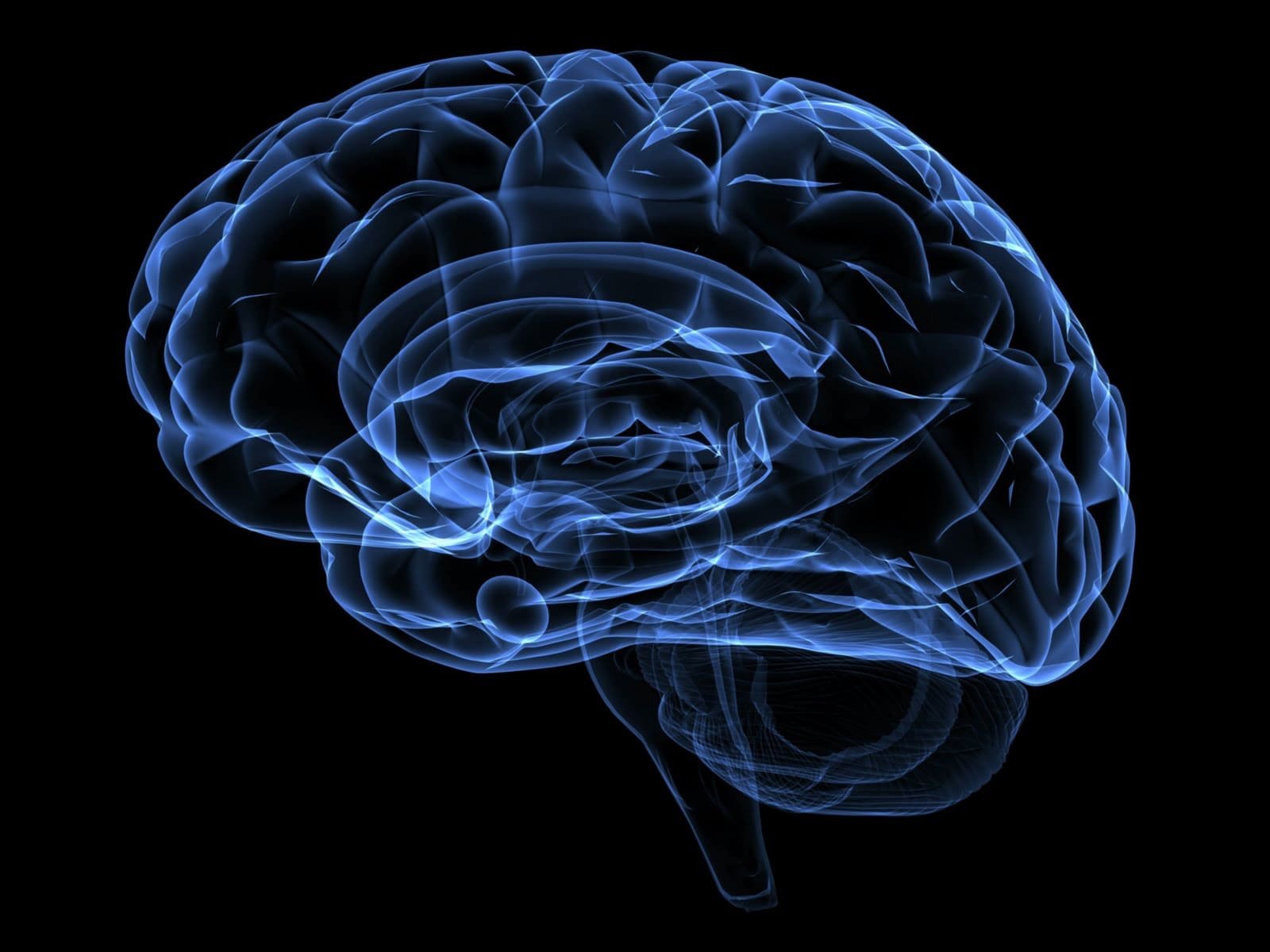
RESEARCH
The primary inspiration behind our research is the observation that emotions color our lives, and even subtle, everyday variations in our emotional experience can alter our thoughts and actions. By uncovering the impact of emotion and affect on cognition, we aim to both enhance our understanding of cognition broadly and provide insights into social processes and psychological disorders.
To address this topic, the lab uses a Human Neuroscience approach, combining insights from sub-disciplines of psychology and neuroscience to understand the mind and brain. Along these lines, we try to let the questions drive our research, not the techniques or traditional definitions of research areas. We have used a number of techniques (behavioral studies, physiological measurements, hormone assays, pharmacology, microbiome assays, brain-lesion studies, functional magnetic resonance imaging, computational modeling) and have worked with a number of collaborators in different domains (social, clinical, and cognitive psychologists, psychiatrists, neuroscientists, neurologists, economists, physicists, computational neuroscientists). It is our hope that having focused questions and a broad approach to answering these questions enhances both the research quality and the relevance and appeal of our work.
There are three overarching themes of the current research in the Phelps Lab.
MECHANISMS OF THREAT CONTROL
When we first started investigating the neural mechanisms of emotion’s influence on learning and memory, there was very little research in humans outlining the neural systems of emotion. Research with non-human animals indicated that the amygdala was a key structure in emotional learning, in particular the acquisition and expression of classical fear or threat conditioning. Our first goal was to determine if the amygdala played a similar role in humans (LaBar et al., 1995; 1998), and if more typical means of emotional learning in humans (verbal instruction and observation) and social learning about others (race attitudes) engaged overlapping neural systems (see Olson & Phelps, 2007; Kubota et al., 2012 for reviews).
Over the last decade or so we have focused on examining how learned fear or threat reactions can be modified and controlled, which has more direct translation to clinical interventions (see Dunsmoor et al., 2015, Neuron for a review). Along these lines, we were the first to examine the neural mechanisms of extinction in humans (Phelps et al., 2004), and also the first lab to demonstrate that behavioral interventions may be able to persistently inhibit defensive responses by targeting memory reconsolidation (Schiller et al., 2010; 2013; Steinfurth et al., 2015). These studies were tightly integrated with collaborative projects in animal models.
We are currently further investigating the mechanisms of reconsolidation (e.g., Steinfurth et al., 2014; Kroes et al., 2017), and are exploring and identifying additional techniques and factors that may enhance, modulate or impair the adaptive, flexible control of threat. These include acute stress (e.g., Raio et al., 2013; 2014; 2017), threat memory generalization (Dunsmoor et al., 2017a; 2017b; Kroes et al., 2017), and cognitive and social factors such as reappraisal-based emotion regulation, verbal instruction and cognitive load (e.g., Delgado et al., 2008; Shurick et al., 2012, Atlas et al., 2017, de Vgood et al., 2018). We are also exploring novel techniques to enhance extinction and prevent maladaptive threat recovery by reducing uncertainty (Dunsmoor et al., 2015), or increasing resilience via stressor controllability and avoidance (e.g., Delgado et al., 2009; Hartley et al., 2014, Boeke et al., 2017). Importantly, this research combines insights from animal models, quantitative learning models, and cognitive and social psychology to determine the range of factors that contribute to the flexible, adaptive control of fear and threat in humans.
Our laboratory also extends this research to collaborative projects with clinical psychologists, most notably researchers at the Center for Anxiety and Related Disorders at Boston University. The goal of this collaborative research is to determine which approaches or techniques might most readily translate to novel and more effective clinical interventions.
EMOTION AND DECISION-MAKING
Our research on threat learning investigates how neutral stimuli acquire an aversive value via pairing with an aversive event. Learning about value through association or feedback is captured in quantitative reinforcement learning models. However, most current research on reinforcement learning examines learning through positive (appetitive or rewarding) associations or feedback. We have taken advantage of quantitative reinforcement learning models in the appetitive domain and examined if learning signals in the brain are similar or different across aversive and appetitive reinforcer types (e.g., Delgado et al., 2008; Li et al., 2011), and if they are similarly influenced by cognitive and social factors (e.g., Delgado et al., 2008, Li et al., 2011; Atlas et al., 2016).
Investigating the links between aversive and appetitive learning and how they motivate action prompted us to delve into research in the discipline of Neuroeconomics. This led to a series of studies in which we attempt to investigate and characterize the behavioral and neural interactions of affect and decision-making (see Phelps et al. 2014, Annual Review in Neuroscience, for a discussion). Our Neuroeconomic research is often conducted in collaboration with Behavioral Economists (Robert Frank, Andrew Schotter, Colin Camerer), Neuroeconomists (Paul Glimcher) or Computational Neuroscientists (Nathaniel Daw), and uses classic behavioral economic tasks and quantitative models of behavior, along with a range of techniques from affective neuroscience. We explore two different means by which affect can influence decisions. The first is based on the premise that the emotional reaction to the choice is a key component of the calculation of subjective value for that choice. The second explores how an affective state that is unrelated and incidental to the choice nevertheless biases the decision.
The primary emotional reaction we have assessed in this first line of research is physiological arousal. Specifically, we have shown that arousal to losses in a risky decision task, but not gains, predicts loss aversion, and that altering arousal through either cognitive emotion regulation or pharmacology reduces loss aversion, as well as amygdala BOLD responses to losses (Sokol-Hessner et al., 2009; 2012; 2016). Importantly, using quantitative models from Behavioral Economics we were able to dissociate the impact of arousal on loss aversion from risk aversion, and found that arousal is unrelated to assessments of risk. More recently, we were able to show that arousal correlates with ambiguity aversion as well (FeldmanHall et al., 2016). We have also been able to specify the role of arousal in intertemporal choice (Lempert et al., 2015; 2016), and demonstrate how altering affective factors through pharmacology or behavioral techniques can change temporal discounting (Lempert et al., 2017; 2018).
The primary affective state we have manipulated in the second line of research assessing the incidental role of affect in decisions is stress, both acute and chronic. Specifically, we have shown that inducing acute stress prior to the decision task may shift one from a model-based to a model-free decision-making strategy (Otto et al., 2013), and both acute and chronic stress may cause one to over-exploit current resources in a foraging task and diminish optimal behavior (Lenow et al., 2017). Interestingly, we also found that acute stress did not impact assessments of either loss aversion or risk sensitivity (Sokol-Hessner et al., 2016)
Finally, in a series of studies we have explored how learning and decision-making are influenced by social factors that may evoke affective responses, including social competition (Delgado et al., 2008), race (Olsson et al., 2005; Stanley et al., 2011; Kubota et al., 2013; Dunsmoor et al., 2016), and assessments of fairness (FeldmanHall et al., 2014). We are also examining how factors such as stress, episodic memory, and generalization of learning influence social decision-making (FeldmanHall et al., 2015; 2018; Murty et al., 2016).
EMOTION AND MEMORY
Another research agenda in our laboratory is to understand how emotion interacts with episodic memory. Our studies in this domain have explored both how emotion enhances some aspects of memory accuracy, but not others, and how emotion influences the subjective sense of vividness and confidence in memory accuracy. To do this, we have explored the role of emotion at all stages of memory formation (attention and perception or encoding, consolidation, retrieval, reconsolidation -- see Sharot & Phelps 2007 for a review).
Our current research in this area examines the relationship between emotion’s influence on subjective judgments of recollection and accuracy for memory details (Hirst et al., 2009; Rimmele, 2011; 2012), as well as how emotional arousal may carry-over to enhance memory for neutral stimuli encoded after an emotional event (Tambini et al., 2017). We have also recently shown that the influence of emotion on episodic memory can extend retroactively to enhance memory for neutral stimuli encoded prior to affective learning about the significance of related stimuli (Dunsmoor et al., 2015; Patil et al., 2016). We interpret these finding as consistent with tag-and-capture models of memory suggested by research with non-human animals, and are currently trying to determine if we can identify a neural signature of memories ‘tagged’ for later enhancement via emotional learning.
Finally, in ongoing research we are examining how altering threat or defensive responses indicative of fear memories through either extinction learning or targeting reconsolidation may influence the enhancement of episodic memory for related stimuli (Dunsmoor et al., 2018; Kroes et al., 2017) or the specificity of those memories (Starita et al., 2019). We are also exploring the influence of lifetime stress or acute stress at different stages of memory formation on later memory accuracy or the expression of different memory systems (Goldfarb et al., 2016; 2017).